HousingWire recently spoke with Mohammad Rashid, Head of Fintech Practice at Tavant, about ways that he believes banks could better utilize AI and and machine learning, thereby improving the customer experience.
FinLedger: How can banks utilize AI and machine learning to better serve the consumer?
Mohammad Rashid: We are in an AI-powered digital era. An era in which every single individual, who is also a potential bank customer, has an ever-increasing digital footprint due to increased access to financial and non-financial transactions through digital pathways. AI and machine learning processing capabilities are the most advanced techniques available today to crunch this growing sea of data and extract meaningful insights on customer financial lifecycle, overall sentiment and predict customer behaviors with accuracy.
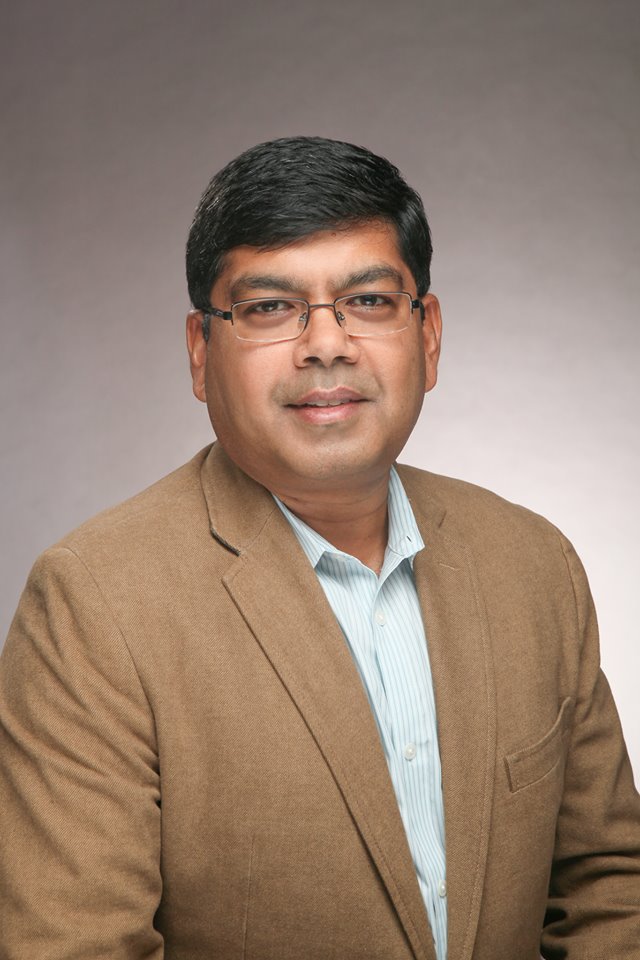
Machine learning and the exponential growth of digitally consumable data for bank customers today allow banks to implement several use cases that were previously difficult or cost-prohibitive to rollout. Some of these use cases include fraud detection, hyper-personalization of the customer journey and automated underwriting.
Understanding customer behavior, sentiment or reaction to a specific product or service can now be understood with more clarity due to the availability of data from a diversity of sources – including social media. Banks can then use these insights to provide these customers with highly tailored or highly contextual advice at the right time.
AI and machine learning technologies can help boost revenues through increased personalization of services to customers (and employees); lower costs through efficiencies generated by higher automation, reduced errors rates, and better resource utilization; and uncover new and previously unrealized opportunities based on an improved ability to process and generate insights from vast troves of data.
FL: What are some trends you are seeing in terms of banks personalizing offerings for the consumer?
The falling cost of data storage and processing capacity (ML models need to crunch very large data sets), increased access to distribution platforms, and increased understanding of the machine learning models makes for an interesting case for financial institutions taking an AI-first approach to understand consumer behavior and create what is called an in-depth 360-degree view or profile of the customer. This allows them to segment their customer base into specific personas and provide targeted services and offerings to each persona.
The digital experience leaders among these financial institutions continuously raise the bar on personalization to the point where they sometimes anticipate customer needs before the customer is aware of them and offer highly contextual and personally tailored services at the right time, through the right channel.
In the first few months of the COVID-19 pandemic, the use of online and mobile banking channels across countries has increased by an estimated 20% to 50% and is expected to continue at this higher level once the pandemic subsides.
The commonly used AI technologies are:
- Intelligent Robotic Process Automation for structured and somewhat complex operational tasks,
- Virtual assistants or conversational interfaces for call center operations and customer service divisions, and
- Machine learning techniques to detect fraud and support underwriting and risk management strategies
FL: What are some challenges financial institutions face when leveraging behavioral analytics?
Most financial institutions have monolithic systems for their core banking, lending and wealth management platforms. These platforms are brittle and inflexible and do not allow for the injection of customer insights, and behavioral analytics gathered from ML models to color their offerings.
Financial institutions may also have traditional operating models that are not amenable to change and need to be rethought to incorporate these just-in-time, contextual offerings.
There is an added factor that a number of these financial institutions are new to AI and machine learning approaches and do not realize that the proof point and efficiency in machine learning may start out slow but then can provide benefits exponentially. Due to the immaturity and sometimes due to the hype surrounding AI and ML, some institutions have unrealistic expectations of their initiatives, and therefore, fail even before they get off the ground.
There are several other challenges that evolving firms will encounter on the capability maturity spectrum and must be ready to address. A sampling of these challenges are as follows:
- Inability to gather large data sets (or gather those data points that are relevant) from customer data, interactions, sentiments and transactions or the inability to enrich such data sets with alternative data such as, for the sake of this conversation, macro and micro-economics data including employment numbers, geolocation data and corresponding demographics.
- A rush to embrace AI and ML models without appropriate due diligence creates a context where financial firms rely on mysterious systems that do not explain or provide a rational underpinning for the critical decisions; they make such as credit decisioning and risk alignment. These approaches may introduce invisible bias into the system. The ability to develop explainable AI layers and making sure that they are providing the right justifications and rationale for these decisions is imperative for a wider and more regulatory compliant adoption into the financial arena.
- Not incorporating MLOps into the operational rollout of the machine learning-based initiative. ML models remain an interesting experiment within the confines of a computational lab without a systematic and methodical production rollout strategy. MLOps is the magic elixir that takes it out of the lab (within the context of a successful business) and introduces the value of AI/ML and that initiative into the enterprise.
FL: How does Tavant help alleviate these challenges?
Tavant has been in the lending and mortgage industry for the last 20 years and has more recently entered the real estate, banking and insurance industries. We understand these domains and can offer guidance on the appropriate AI and machine learning use cases that are relevant, providing quick wins for banks.
Tavant has a seasoned and dedicated team of data scientists and data engineers that comprise its AI and analytics practice. Tavant has also developed a number of products that are ML-based as part of its VELOX suite of products, such as Affordability Analyzer for matching borrowers to the right-sized home and the most appropriate loan product and program to minimize future defaults and financial distress. Tavant’s AI&A team also developed a portfolio defense product that helps banks retain their customers but predicting which of these customers may “churn” away from the bank’s portfolio. Tavant also develops custom AI and machine learning solutions for several clients within the Financial industry helping such customers develop, design, deploy, maintain and enhance advanced ML models.
Given the experience and provenance mentioned above, Tavant can help banks with the overall approach, strategy, design, development/execution and production rollout (scaling and operations readiness), as well as the continuous maintenance and enhancements that are required for such models:
- Approach and Strategy: Identify and develop the right use cases. Set the right expectations. Provide a roadmap for training and a target operating model based on the business, technology and operational assessment.
- Design, Development and Execution: Help execute with our domain experts, technical experts, and data scientists, and engineers. Help in developing models and conducting data analytics, as well as building out the right data structure and dashboards.
- Production Rollout (Scaling and Operations Readiness): Analyze the right tech stack and distribution strategies. Help develop the right and meaningful dashboards based on the functional role. Help organizations roll out the use cases at an enterprise level. Implement MLOps strategies so that proof of concepts (PoCs) are evolved from the lab setting to the enterprise context and can provide the lift that is required for business operations to be successful.